News
Precision in battery recycling: RHINOCEROS project’s Disassembly Process Plan leaves no room for errors
30/04/2025
Researchers at the Faculty of Engineering and Science within the University of Agder [UiA] have developed a digital simulator along with an algorithm that automatically generates the Disassembly Process Plan [DPP] for batteries. The algorithm is built to select autonomously the most suitable machine to execute each disassembly operation, along with the corresponding toolkit. Beyond automating the DPP, this technological breakthrough promises to reduce the total disassembly process duration.
With the electrification of mobility, the upcoming wave of e-waste will be hard to deal with. In general terms, electrical waste is shredded in bulk before sorting and reprocessing. But lithium-ion batteries [LiBs], the types used in EVs, are inflammable and request careful handling. Moreover, shredding lots of different types of e-waste simultaneously inevitably results in contamination. Separating components before shredding would yield greater levels of purity, even allowing various components, such as cathodes, to be reused in their entirety. Dismantling batteries is a dangerous operation due to the risk of fire or explosion. Nonetheless, this process typically involves manual labour to remove the casing and separating the internal components – electrodes, electrolyte, cabling and separators.
Within the RHINOCEROS project, the UiA is responsible for developing an automated system for characterising battery state, discharging via the grid and dismantling for reuse or recycling. Their ultimate goal is to reduce the operational duration and to improve resource utilisation. An important phase of their work lies in the development of a Disassembly Process Plan – shortly DPP, which features a disassembly sequence plan [DSP] and an algorithm that can establish autonomously the most suitable equipment for each disassembly operation. To avoid several rounds of unsuccessful trials, researchers have firstly created a digital simulator where they already tested the algorithm generating the DPP.
Trained to use time and resources efficiently
The use of a simulation environment offers a safe and cost-effective way for researchers to test and refine the algorithm in a controlled context. Beyond safety and resource optimisation, simulators facilitate also scalability and reproducibility. The algorithm is trained to select the most suitable toolkit and calculate optimal tool change sequences, which reduces the overall disassembly process duration. Moreover, it also features integrated data that allow it to verify beforehand compatibilities between requested tools and machine capabilities. By determining optimal disassembly sequences and tool allocation, the DPP reduces operational costs through more efficient equipment usage.
Closer look at the technical specifications of the DPP developed in RHINOCEROS
From a technical point of view, the digital simulator, built on a foundation of Python and NumPy, tracks job progress, machine availability, tool states and temporal dependencies. Researchers applied various Reinforcement Learning (RL) algorithms, including Proximal Policy Optimisation (PPO), Policy Gradient (PG), Advantage Actor-Critic (A2C) and Asynchronous Advantage Actor-Critic (A3C), to solve the Job Shop Scheduling Problem (JSSP). The PPO algorithm, in particular, has demonstrated superior performance compared to other algorithms and state-of-the-art (SoA) solutions. The simulator is using datasets similar to the battery disassembling problem, mimicking real use case scenarios.
In a recent development, researchers have introduced an innovative approach called QTM (Q-learning with Tsetlin Machines) to improve scheduling algorithms. This new method combines the pattern recognition abilities of Tsetlin Machines (TM) with the decision-making strengths of Q-learning. The QTM approach uses a sophisticated reward system to balance the completion of tasks, minimize the time taken, and optimise the overall schedule. The TM component excels at identifying patterns in scheduling scenarios by analysing key features of jobs and operations. Meanwhile, the Q-learning framework provides a foundation for learning through a process of trial and error, gradually improving decisions over time.
The DPP developed in the RHINOCEROS project features PPO, QTM and classic deep Q-learning.
Impact on the real-world industrial applications
The DPP brings significant implications for real-world applications, particularly in industries that prioritise efficiency and sustainability. In EV battery recycling, it optimises the disassembly sequence, enhancing efficiency. It is also applicable to electronics WEEE recycling, where complex assemblies require precise disassembly. The DPP can integrate into large industrial scales, enabling the reuse of valuable components and supporting circular business models.
Automation integrators and robotic system developers benefit from the DPP’s computational framework, which models tool-changing operations and associated costs, optimising robotic movements and tool selection. Academic institutions can use the DPP to study broader questions in automated planning and robotic intelligence, providing a well-defined problem structure for testing new algorithms and heuristics.
For industrial research labs, the DPP offers a framework to develop specialised applications across different product categories, evaluate alternative product designs for end-of-life processing efficiency and simulate different disassembly strategies before physical implementation, reducing development costs and accelerating innovation cycles in recycling technologies.
Future developments for the Disassembly Process Plan
Plans for the DPP foresee its integration into a comprehensive digital twin of the manufacturing environment. Before its demonstration in a real-world setup, this digital twin operating with configurable tool capabilities and interdependent job sequences, will have to pass thorough testing in a simulated environment.
© Visual: University of Agder [UiA]
News
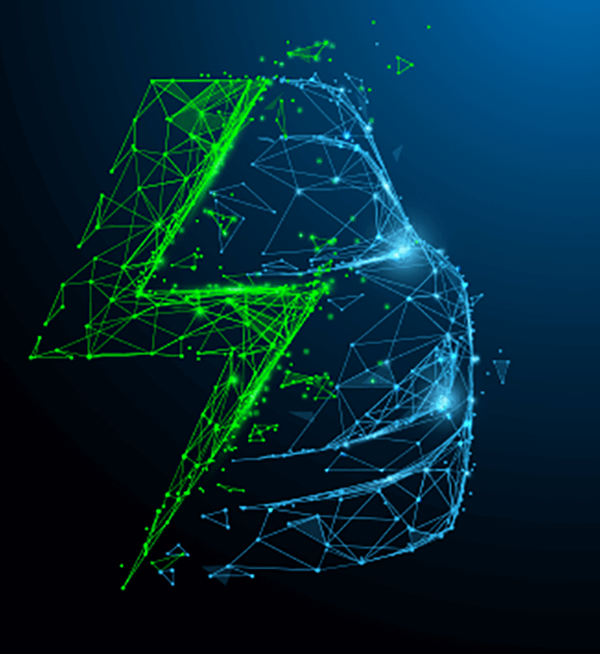
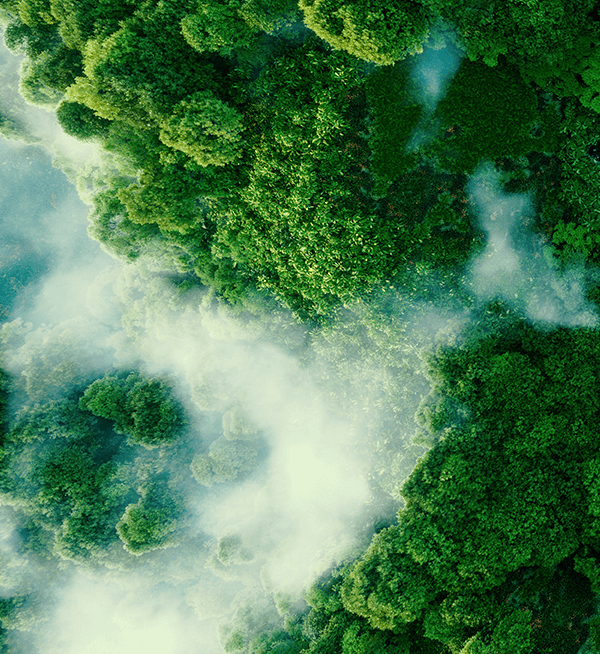